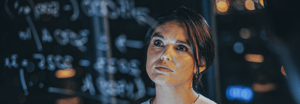
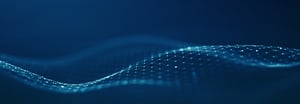
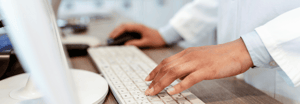
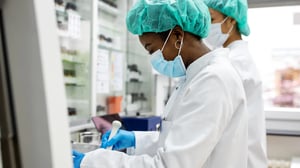
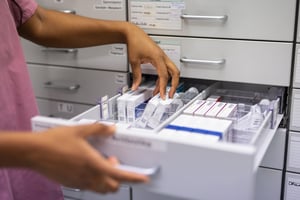
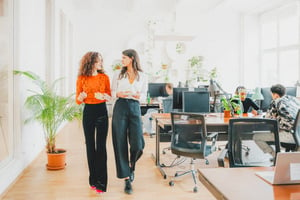
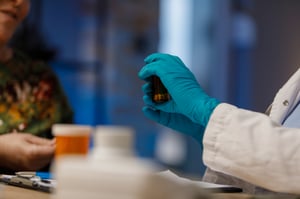
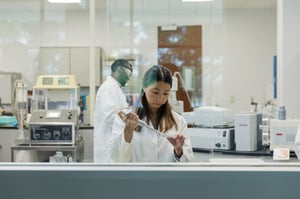
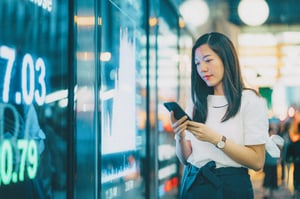
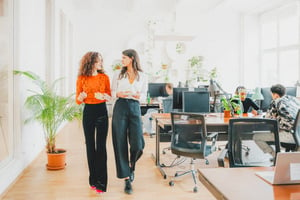
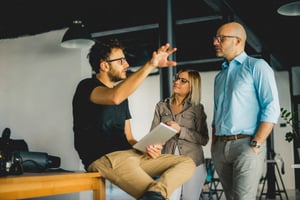
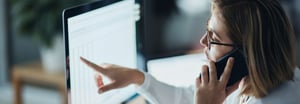
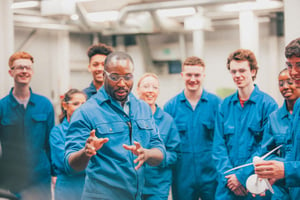
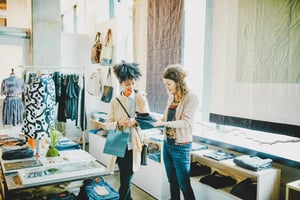
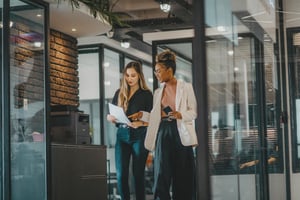
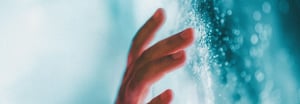
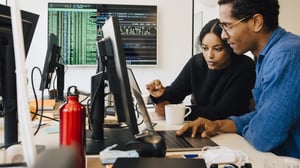
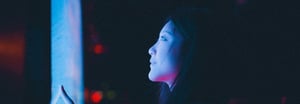
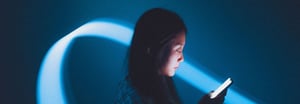
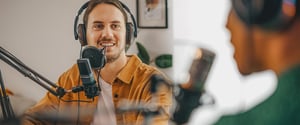
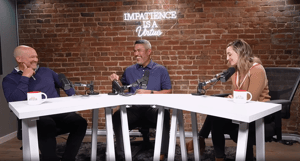
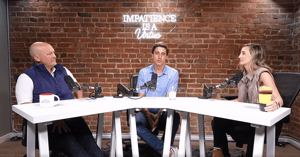
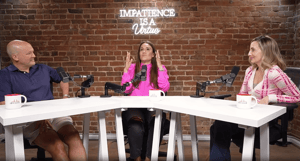
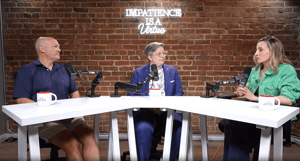
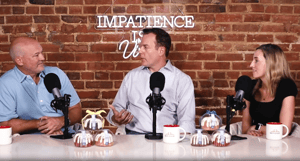
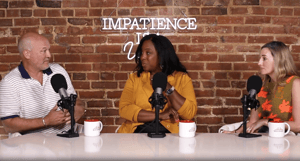
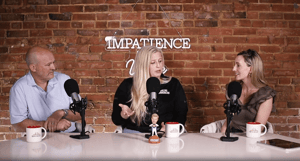
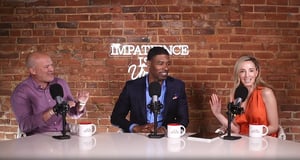
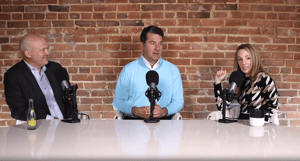
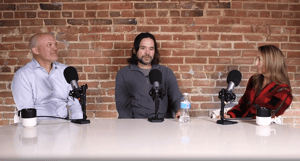
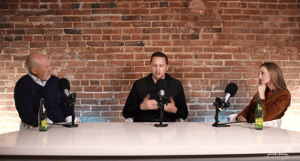
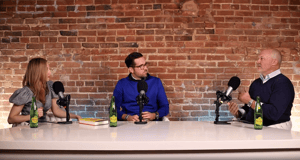
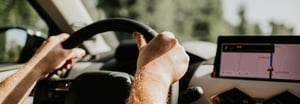
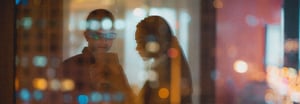
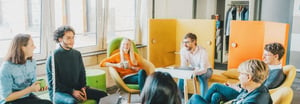
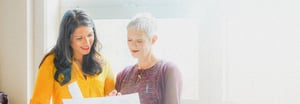
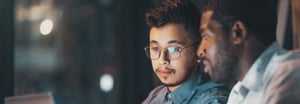
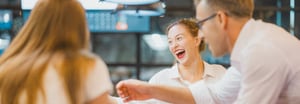
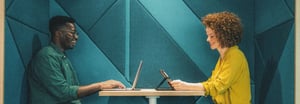
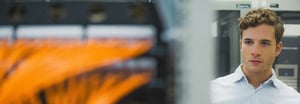
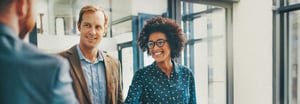
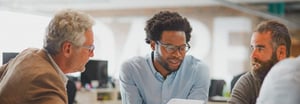
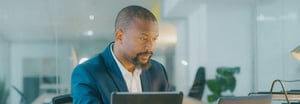
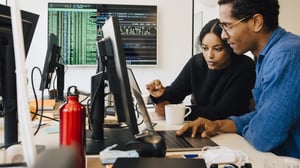
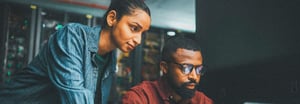
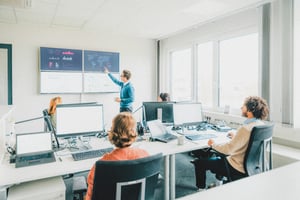
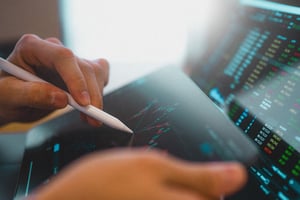
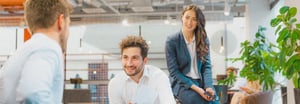
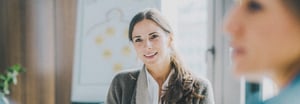
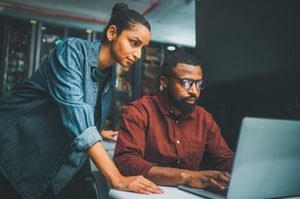
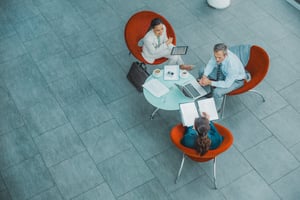
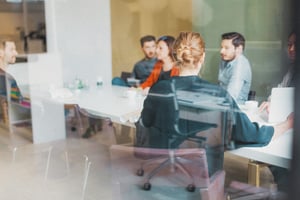
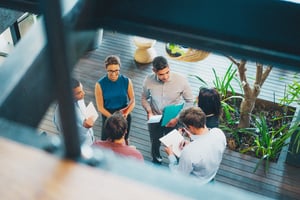
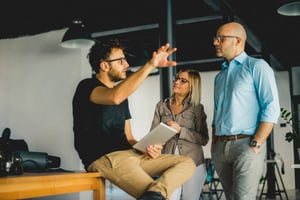
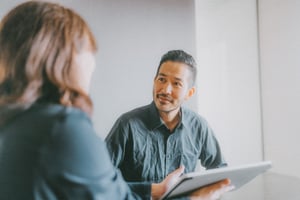
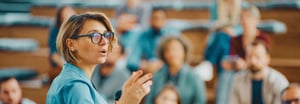
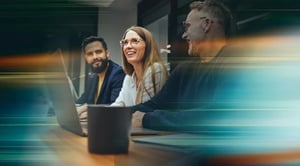
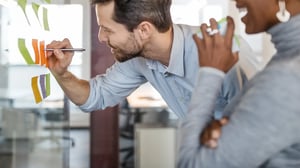
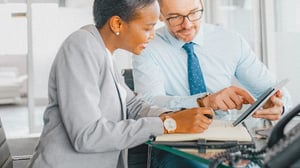
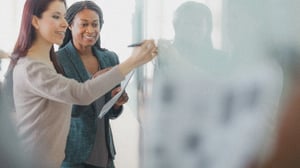
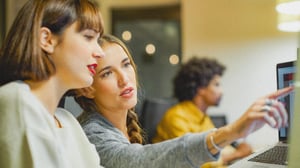
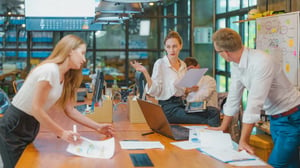
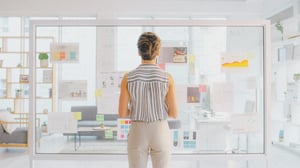
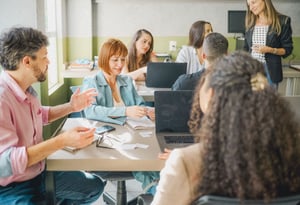
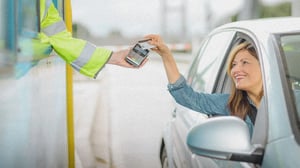
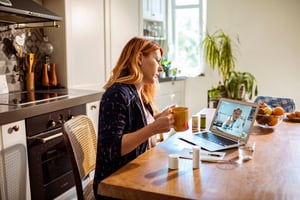
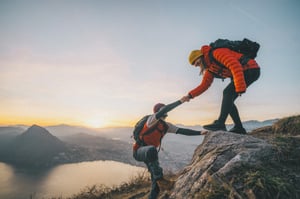
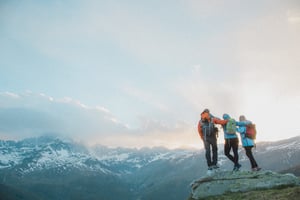
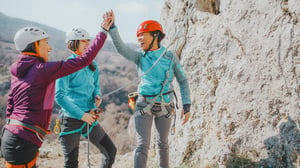
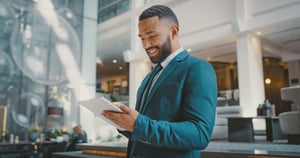
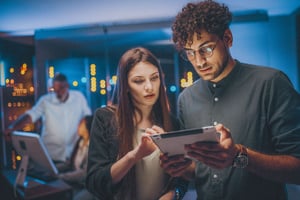
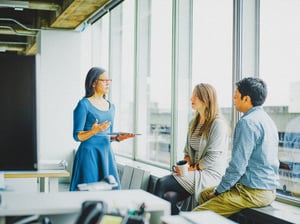
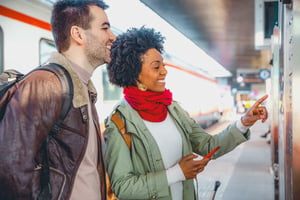
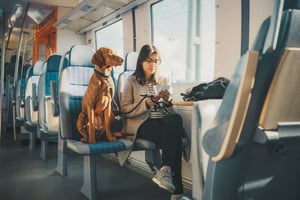
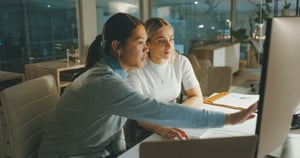
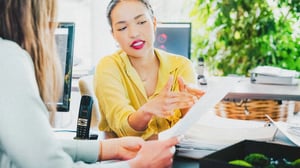
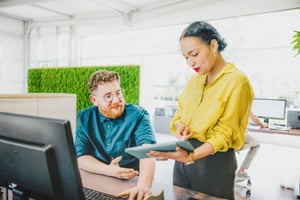
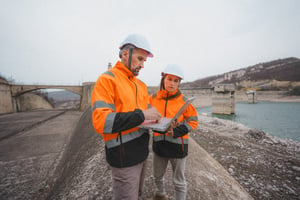
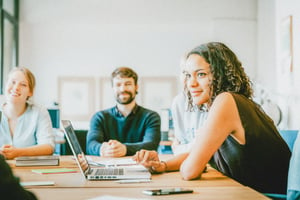
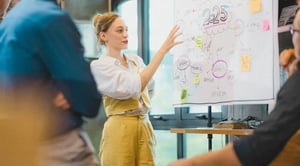
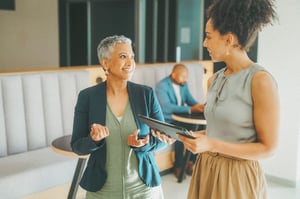
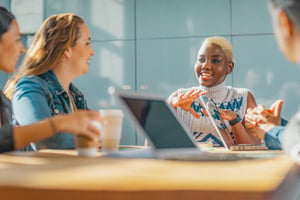
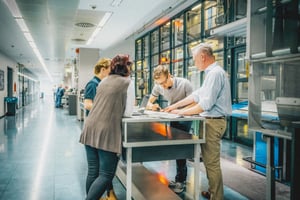
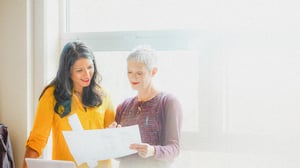
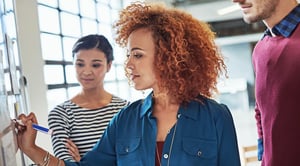
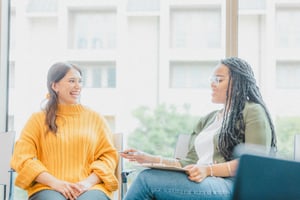
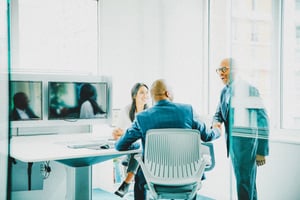
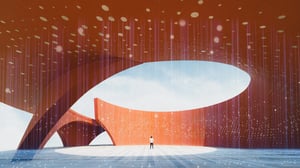
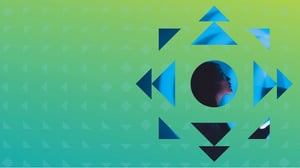
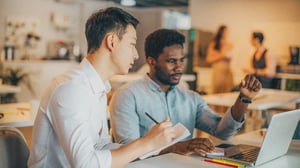
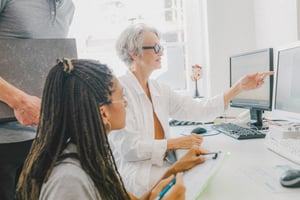
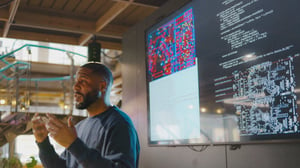
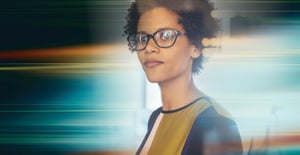
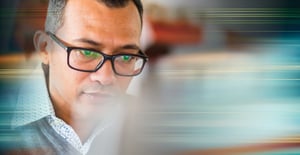
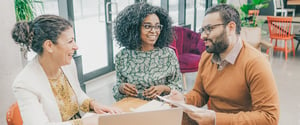
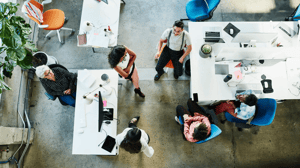
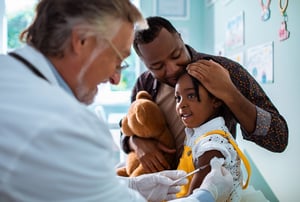
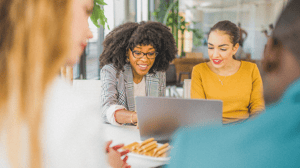
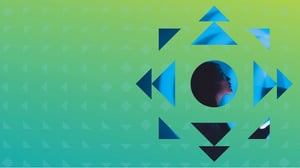
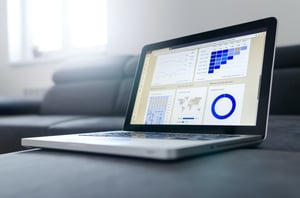
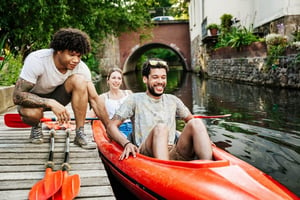
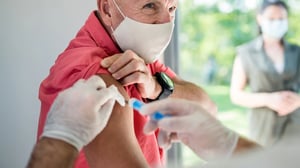
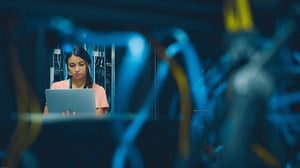
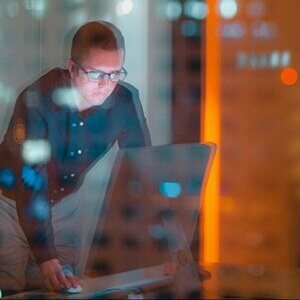
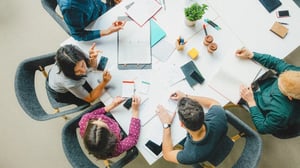
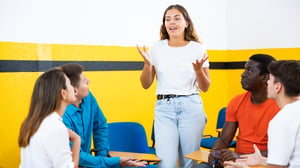
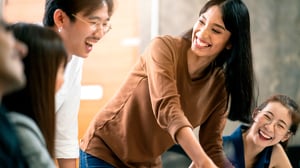
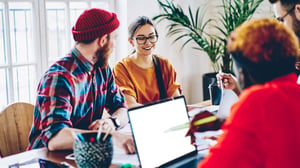
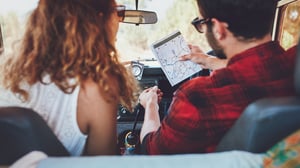
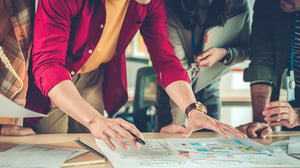
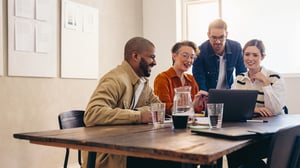
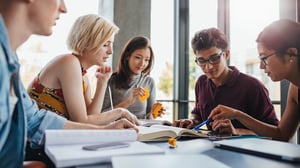
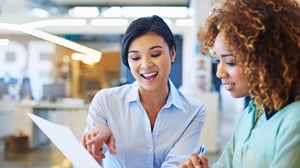
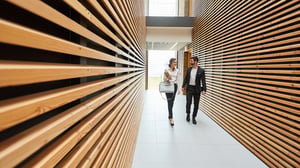
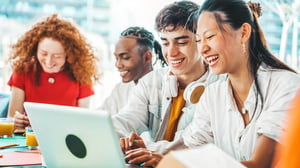
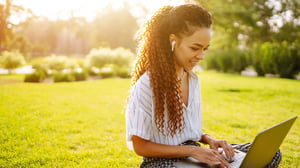
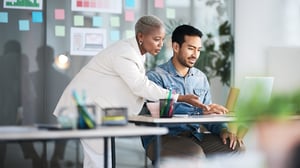
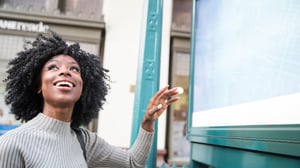
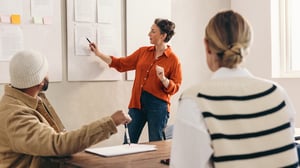
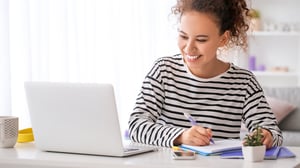
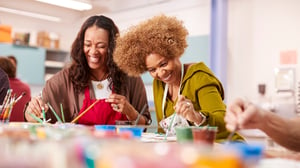
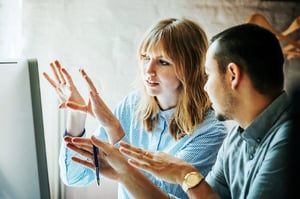
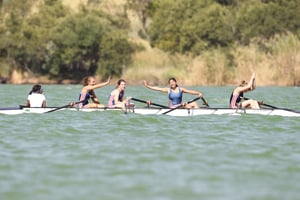
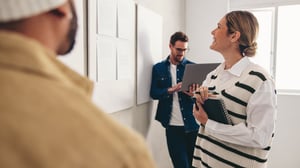
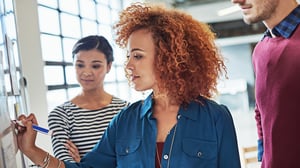
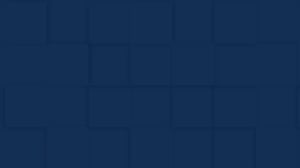
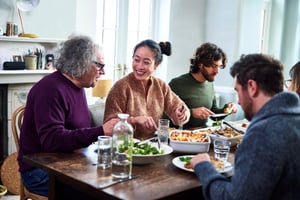
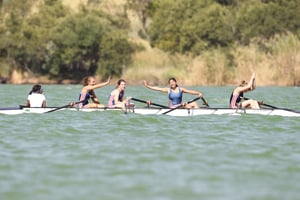
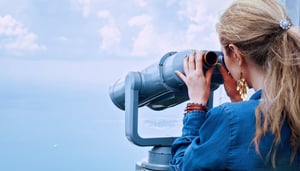
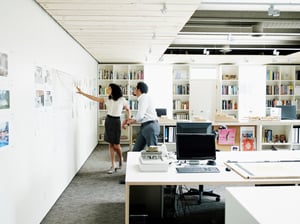
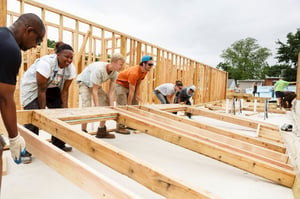
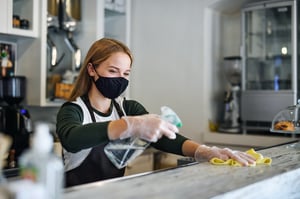
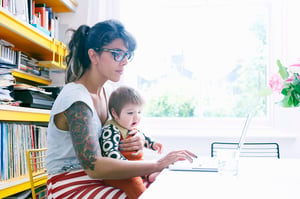
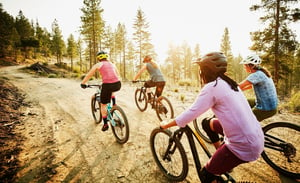
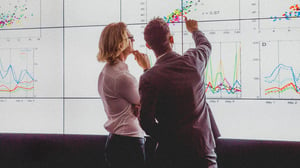
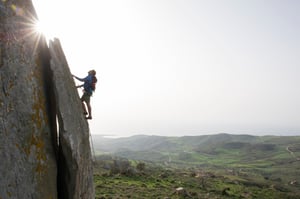
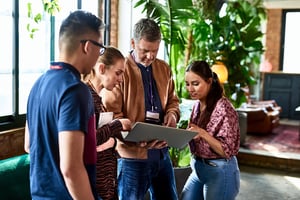
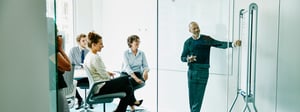
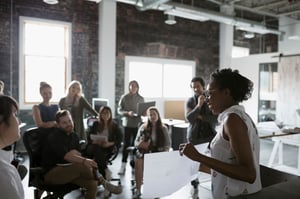
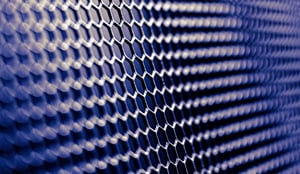
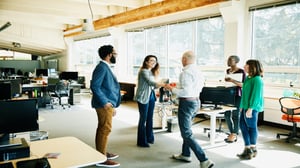
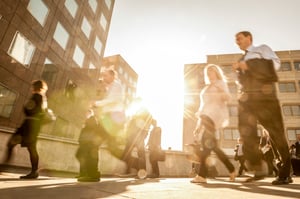
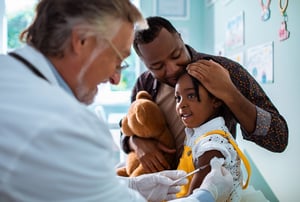
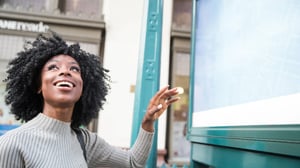
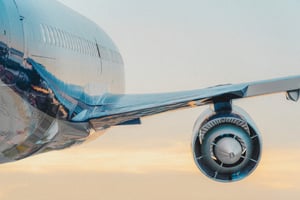
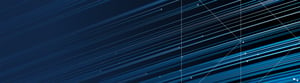
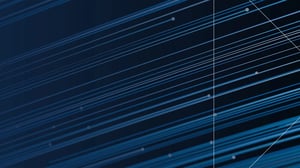
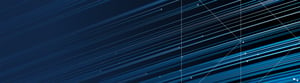
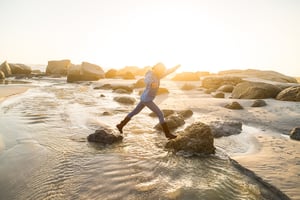
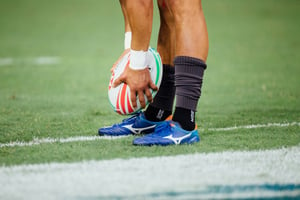
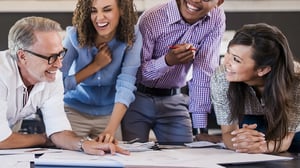
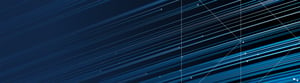
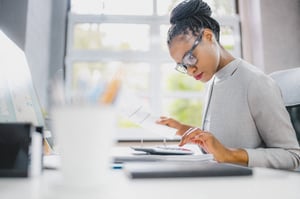
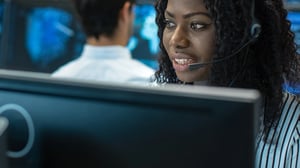
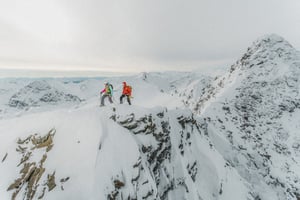
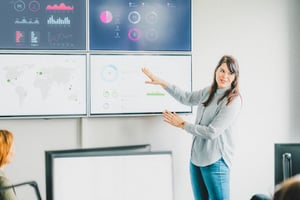
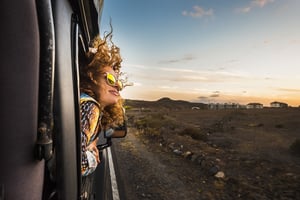
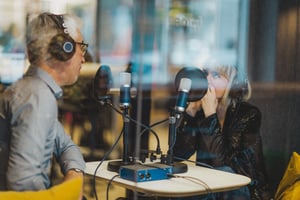
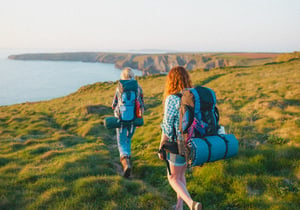
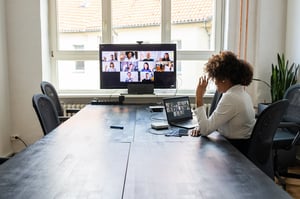
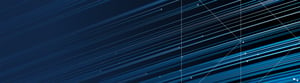
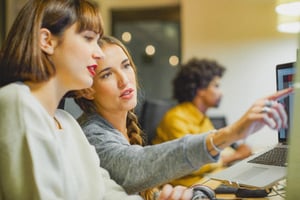
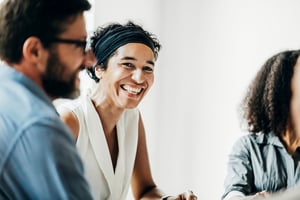
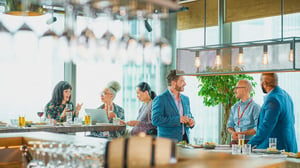
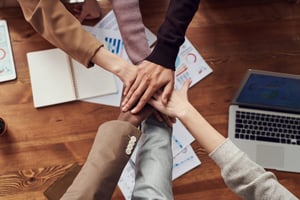
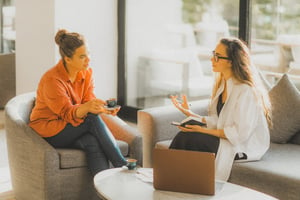
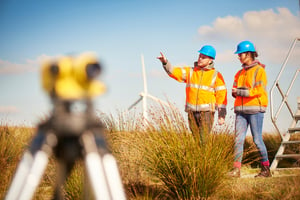
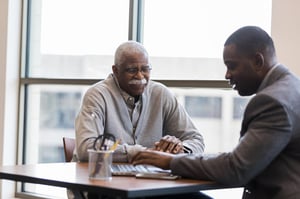
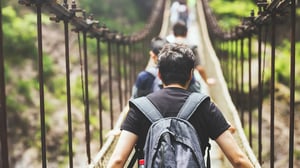
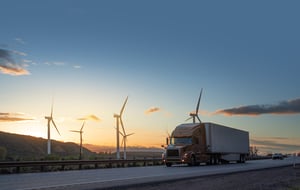
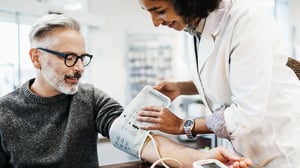
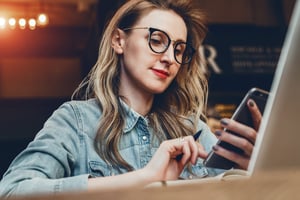
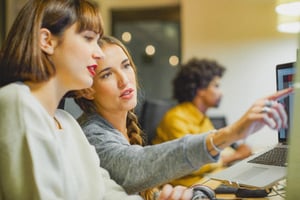
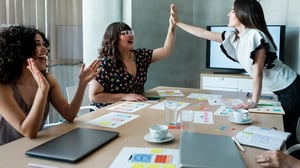
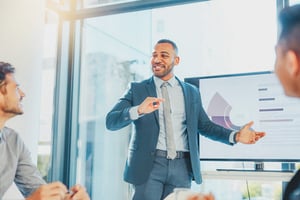
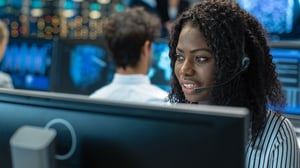
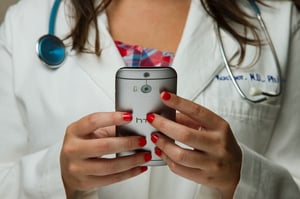
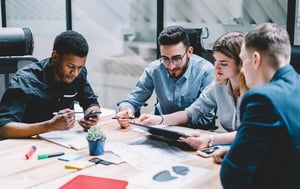
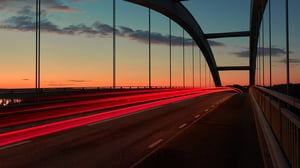
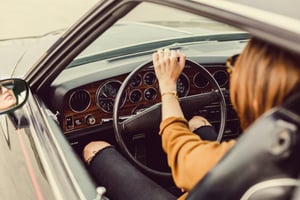
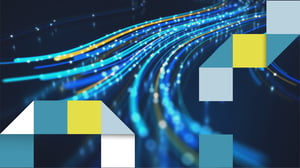
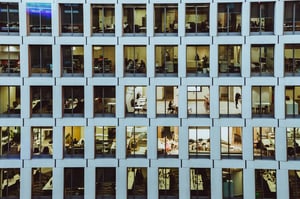
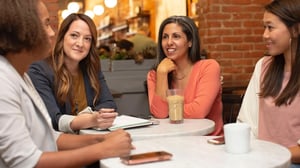
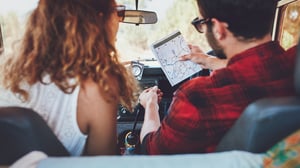
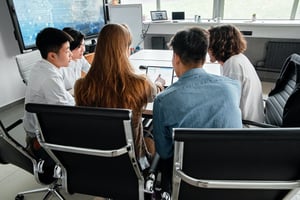
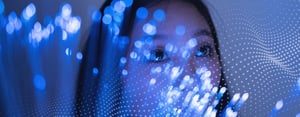
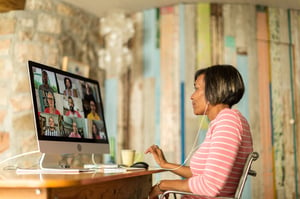
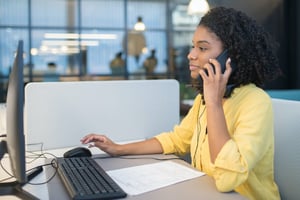
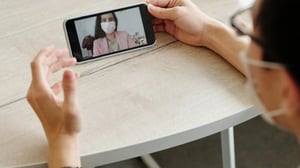
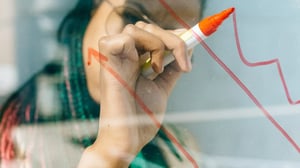
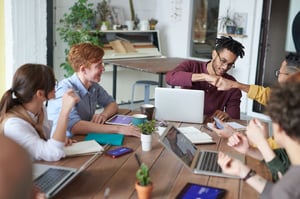
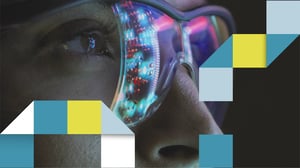
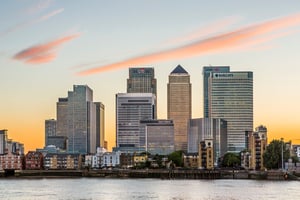
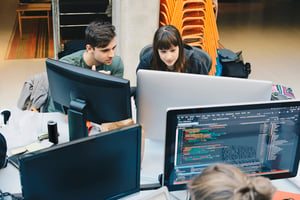
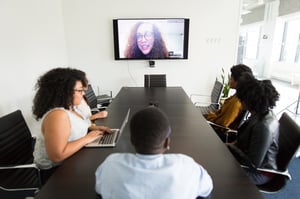
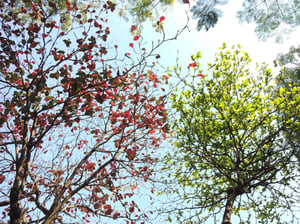
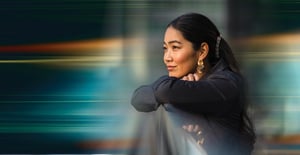
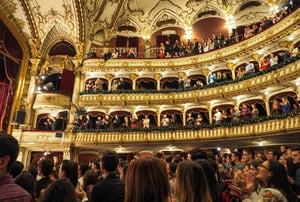
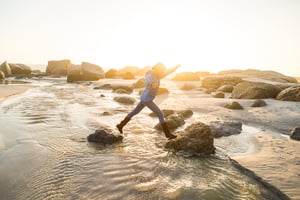
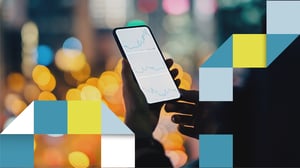
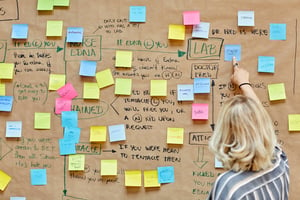
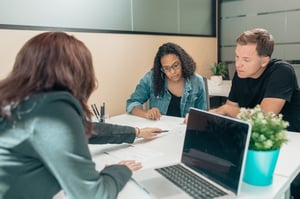
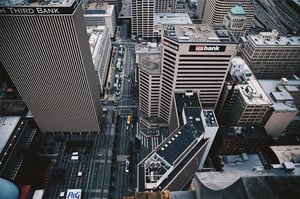
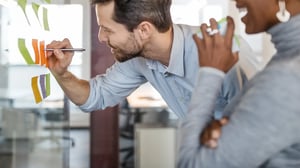
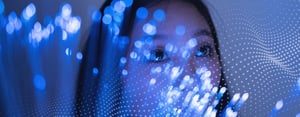
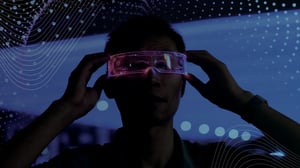
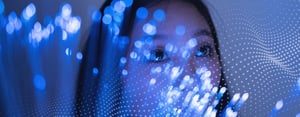
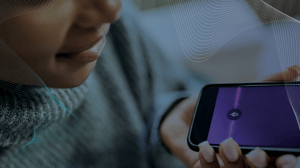
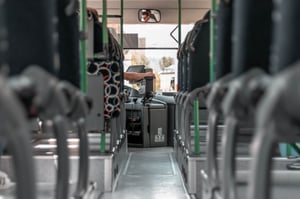
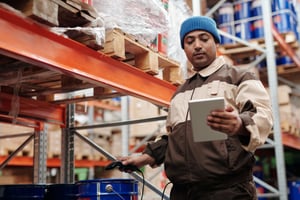
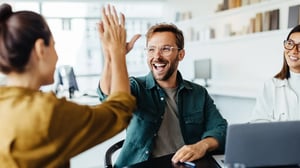
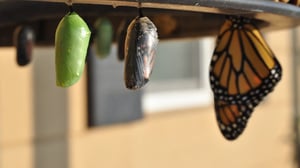
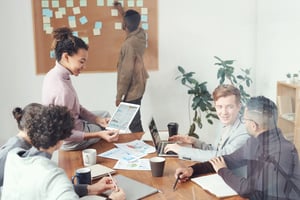
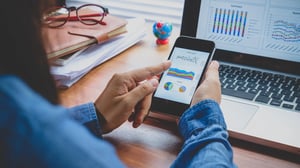
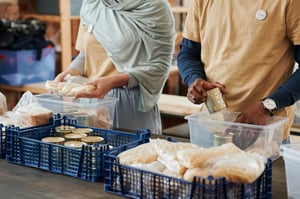
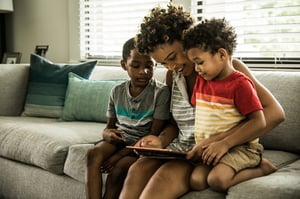
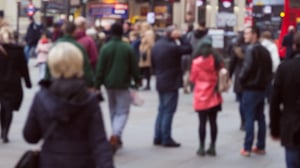
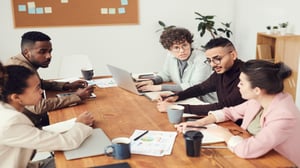
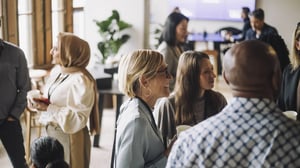
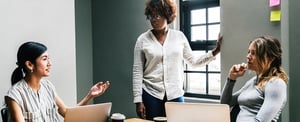
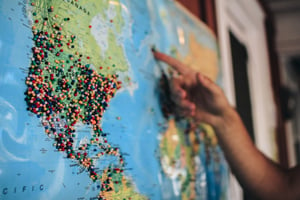
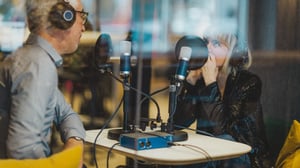
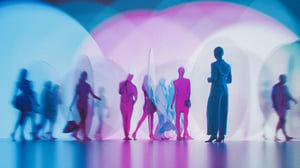
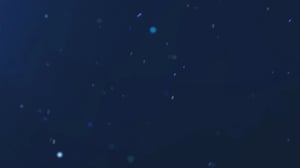
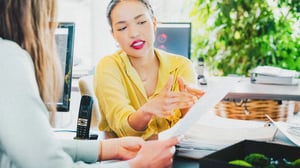
The Bridge, a rapidly growing full-service technology and data consultancy, joins North Highland furthering our digital, tech and AI expertise.
Our latest report informed by 500 business leaders reveals how to unlock AI's true value.
North Highland's AI expert shares a future where hyper-personalization is made possible for everyone.
Are you ready to work with changemakers who bring fresh perspectives, global experience, and a passion for solving problems?
225 results found